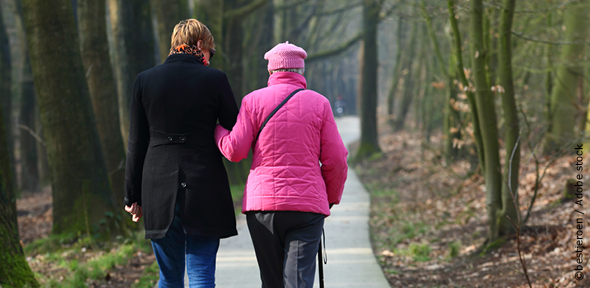
By Dr Abhirup Ghosh
October 2022
About 1 in 9 Americans aged 65 and above has Alzheimer’s Disease (AD) [1]. The UK is currently estimated to have 850k dementia patients associated with £23 billion economic cost, which is predicted to triple by 2040 [2]. Despite its prevalence and the fact that progressive decline in everyday activities is central to AD, current diagnostic tests are neither scalable nor measure changes in daily activities. For example, biomarker-based tests (ligand-PET, CSF assays) are not scalable to population-level usage because of invasiveness, high cost and low availability.
We address some of these issues by investigating everyday navigation. Spatial navigation is one of the first cognitive abilities to be affected in AD: the disease pathology appears early in the brain’s navigation centre (i.e., medial temporal lobe and parietal structures). Previous studies using Virtual Reality found that navigation features help to identify preclinical AD and can predict the clinical progression too.
However, the effect of AD on real-world navigation performance is less understood. We have make strides in this direction by building a machine learning based diagnostic classifier to separate controls from the AD patients using outdoor mobility data only [3]. The key contribution is the identification of distilled mobility features of individuals, extracted from their mobility data. Our findings would ideally be used to build monitoring apps on smartphones or wearables. These devices are already in widespread general use which will allow low-cost application at scale, further passive sensing rather than active testing minimises user involvement, aiding compliance and adherence.
About our study
We explored the real-life outdoor navigation patterns of 15 community-dwelling AD patients and 18 age and gender-matched healthy controls tracked for 15 days using GPS trackers. GPS locations are inherently noisy because of localization errors, but our analysis does not need precise locations. The data may, however, be convoluted by the fact that the patients sometimes were accompanied by their carers; the carer may have influence on the mobility characteristics. We extracted features from the trajectory segments where a person returns to the same location within 1 and 20 min and travels at least a certain length.
Our main findings are as follows:
- Patients’ movements were confined to a spatially smaller region than the ones of controls;
- Patients also followed the same segment route more often than the controls;
- Further, the patients remained closer to their home compared to controls.
We further explored connectivity of visited locations using graph-based features. The rationale for this approach is based on the recent discovery that the hippocampus-based cognitive map can be modelled in terms of graph metrics. As hippocampal degeneration is a central feature of AD, these measures are interesting. We used centrality measures from complex networks and found that the patients typically have a few fixed places from which they go to other places. These features help in diagnostic classification even when the segments with accompanied movements are kept.
Our initial results are exciting although limited availability of data from the relatively short evaluation window did not allow the construction of effective analysis, especially with graphs. We hope future studies at scale and longitudinal AD cohorts will allow the research community to explore the true potential of these techniques.